Blogs

Highlighting Serverless Smarts at re:Invent 2023
Quiz-Takers Return Again and Again to Prove Their Serverless Knowledge
This past November, the Cloudtech team attended AWS re:Invent, the premier AWS customer event held in Las Vegas every year. Along with meeting customers and connecting with AWS teams, Cloudtech also sponsored the event with a booth at the re:Invent expo.
With a goal of engaging our re:Invent booth visitors and educating them on our mission to solve data problems with serverless technologies, we created our Serverless Smarts quiz. The quiz, powered by AWS, asked users to answer five questions about AWS serverless technologies, and scored quiz-takers based on accuracy and speed at which they answered the questions. Paired with a claw machine to award quiz-takers with a chance to win prizes, we saw increased interest in our booth from technical attendees ranging from CTOs to DevOps engineers.
But how did we do it? Read more below to see how we developed the quiz, the data we gathered, and key takeaways we’ll build on for re:Invent next year.
What We Built
Designed by our Principal Cloud Solutions Architect, the Serverless Smarts quiz was populated with 250 questions with four possible answers each, ranging in difficulty to assess the quiz-taker’s knowledge of AWS serverless technologies and related solutions. When a user would take the quiz, they would be presented with five questions from the database randomly, given 30 seconds to answer each, and the speed and accuracy of their answers would determine their overall score. This quiz was built in a way that could be adjusted in real-time, meaning we could react to customer feedback and outcomes if the quiz was too difficult or we weren’t seeing enough variance on the leaderboard. Our goal was to continually make improvements to give the quiz-taker the best experience possible.
The quiz application's architecture leveraged serverless technologies for efficiency and scalability. The backend consisted of AWS Lambda functions, orchestrated behind an API Gateway and further secured by CloudFront. The frontend utilized static web pages hosted on S3, also behind CloudFront. DynamoDB served as the serverless database, enabling real-time updates to the leaderboard through WebSocket APIs triggered by DynamoDB streams. The deployment was streamlined using the SAM template.
Please see the Quiz Architecture below:
What We Saw in the Data
As soon as re:Invent wrapped, we dived right into the data to extract insights. Our findings are summarized below:
- Quiz and Quiz Again: The quiz was popular with repeat quiz-takers! With a total number of 1,298 unique quiz-takers and 3,627 quizzes completed, we saw an average of 2.75 quiz completions per user. Quiz-takers were intent on beating their score and showing up on the leaderboard, and we often had people at our booth taking the quiz multiple times in one day to try to out-do their past scores. It was so fun to cheer them on throughout the week.
- Everyone's a Winner: Serverless experts battled it out on the leaderboard. After just one day, our leaderboard was full of scores over 1,000, with the highest score at the end of the week being 1,050. We saw an average quiz score of 610, higher than the required 600 score to receive our Serverless Smarts credential badge. And even though we had a handful of quiz-takers score 0, everyone who took the quiz got to play our claw machine, so it was a win all around!
- Speed Matters: We saw quiz-takers soar above the pressure of answering our quiz questions quickly, knowing answers were scored on speed as well as accuracy. The average amount of time it took to complete the quiz was 1-2 minutes. We saw this time speed up as quiz-takers were working hard and fast to make it to the leaderboard, too.
- AWS Proved their Serverless Chops: As leaders in serverless computing and data management, AWS team members showed up in a big way. We had 118 people from AWS take our quiz, with an average score of 636 - 26 points above the average - truly showcasing their knowledge and expertise for their customers.
- We Made A Lot of New Friends: We had quiz-takers representing 794 businesses and organizations - a truly wide-ranging activity connecting with so many re:Invent attendees. Deloitte and IBM showed the most participation outside of AWS - I sure hope you all went back home and compared scores to showcase who reigns serverless supreme in your organizations!
Please see our Serverless Smarts Leaderboard below
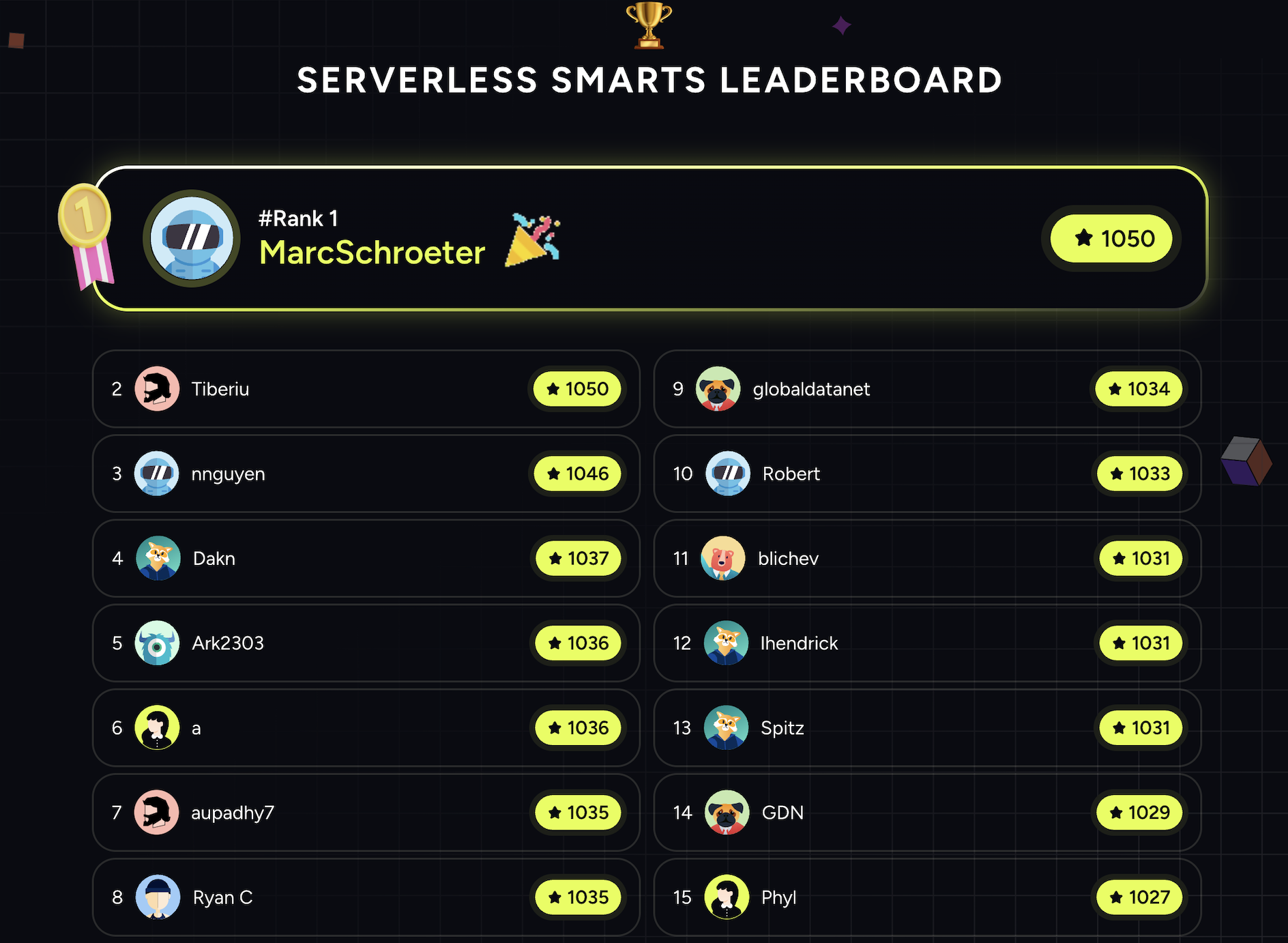
What We Learned
Over the course of re:Invent, and our four days at our booth in the expo hall, our team gathered a variety of learnings. We proved (to ourselves) that we can create engaging and fun applications to give customers an experience they want to take with them.
We also learned that challenging our technology team to work together and injecting some fun and creativity into their building process combined with the power of AWS serverless products can deliver results for our customers.
Finally, we learned the value of thinking outside the box to deliver for customers is the key to long term success.
Conclusion
re:Invent 2023 was a success, not only in connecting directly with AWS customers, but also in learning how others in the industry are leveraging serverless technologies. All of this information helps Cloudtech solidify its approach as an exclusive AWS Partner and serverless implementation provider.
If you want to hear more about how Cloudtech helps businesses solve data problems with AWS serverless technologies, please connect with us - we would love to talk with you!
And we can’t wait until re:Invent 2024. See you there!
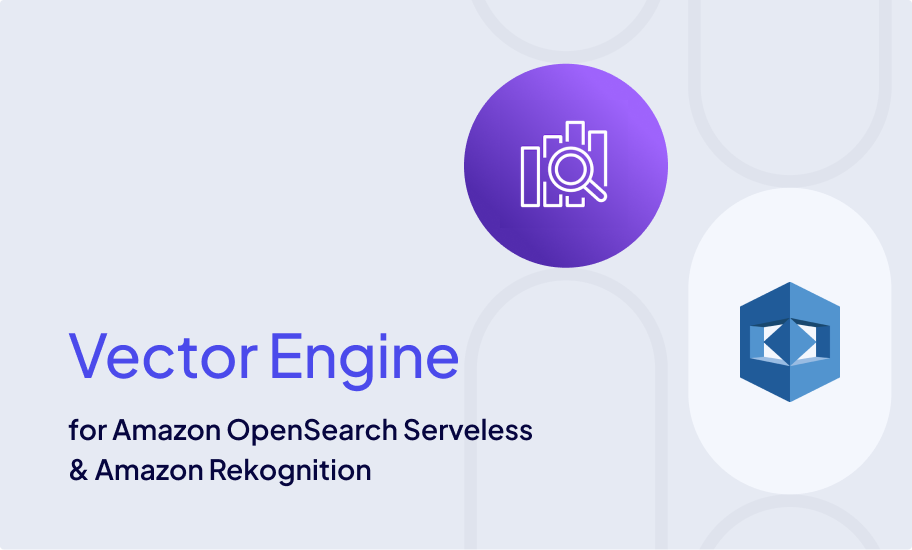
Enhancing Image Search with the Vector Engine for Amazon OpenSearch Serverless and Amazon Rekognition
Introduction
In today's fast-paced, high-tech landscape, the way businesses handle the discovery and utilization of their digital media assets can have a huge impact on their advertising, e-commerce, and content creation. The importance and demand for intelligent and accurate digital media asset searches is essential and has fueled businesses to be more innovative in how those assets are stored and searched, to meet the needs of their customers. Addressing both customers’ needs, and overall business needs of efficient asset search can be met by leveraging cloud computing and the cutting-edge prowess of artificial intelligence (AI) technologies.
Use Case Scenario
Now, let's dive right into a real-life scenario. An asset management company has an extensive library of digital image assets. Currently, their clients have no easy way to search for images based on embedded objects and content in the images. The company’s main objective is to provide an intelligent and accurate retrieval solution which will allow their clients to search based on embedded objects and content. So, to satisfy this objective, we introduce a formidable duo: the vector engine for Amazon OpenSearch Serverless, along with Amazon Rekognition. The combined strengths of Amazon Rekognition and OpenSearch Serverless will provide intelligent and accurate digital image search capabilities that will meet the company’s objective.
Architecture
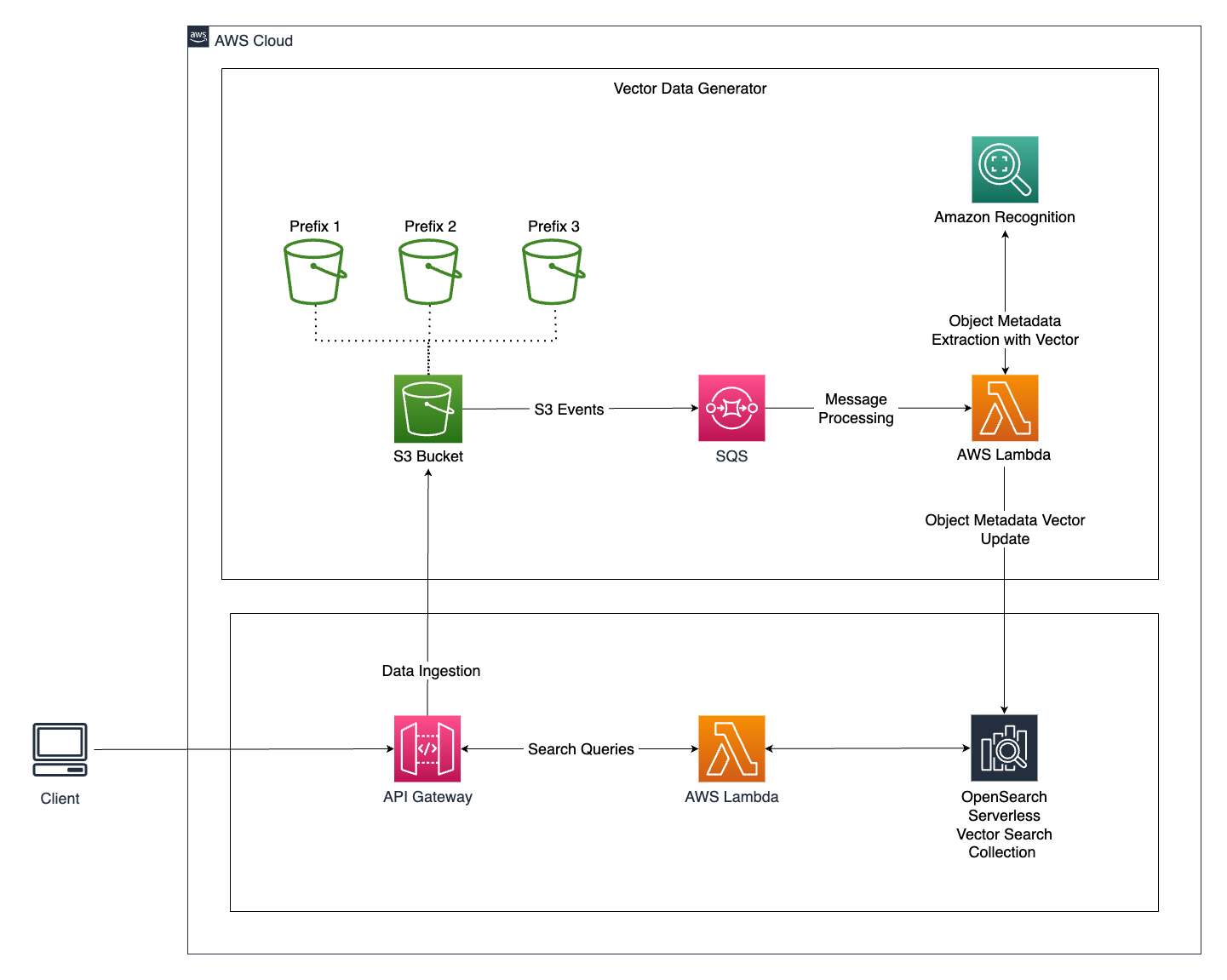
Architecture Overview
The architecture for this intelligent image search system consists of several key components that work together to deliver a smooth and responsive user experience. Let's take a closer look:
Vector engine for Amazon OpenSearch Serverless:
- The vector engine for OpenSearch Serverless serves as the core component for vector data storage and retrieval, allowing for highly efficient and scalable search operations.
Vector Data Generation:
- When a user uploads a new image to the application, the image is stored in an Amazon S3 Bucket.
- S3 event notifications are used to send events to an SQS Queue, which acts as a message processing system.
- The SQS Queue triggers a Lambda Function, which handles further processing. This approach ensures system resilience during traffic spikes by moderating the traffic to the Lambda function.
- The Lambda Function performs the following operations:
- Extracts metadata from images using Amazon Rekognition's `detect_labels` API call.
- Creates vector embeddings for the labels extracted from the image.
- Stores the vector data embeddings into the OpenSearch Vector Search Collection in a serverless manner.
- Labels are identified and marked as tags, which are then assigned to .jpeg formatted images.
Query the Search Engine:
- Users search for digital images within the application by specifying query parameters.
- The application queries the OpenSearch Vector Search Collection with these parameters.
- The Lambda Function then performs the search operation within the OpenSearch Vector Search Collection, retrieving images based on the entities used as metadata.
Advantages of Using the Vector Engine for Amazon OpenSearch Serverless
The choice to utilize the OpenSearch Vector Search Collection as a vector database for this use case offers significant advantages:
- Usability: Amazon OpenSearch Service provides a user-friendly experience, making it easier to set up and manage the vector search system.
- Scalability: The serverless architecture allows the system to scale automatically based on demand. This means that during high-traffic periods, the system can seamlessly handle increased loads without manual intervention.
- Availability: The managed AI/ML services provided by AWS ensure high availability, reducing the risk of service interruptions.
- Interoperability: OpenSearch's search features enhance the overall search experience by providing flexible query capabilities.
- Security: Leveraging AWS services ensures robust security protocols, helping protect sensitive data.
- Operational Efficiency: The serverless approach eliminates the need for manual provisioning, configuration, and tuning of clusters, streamlining operations.
- Flexible Pricing: The pay-as-you-go pricing model is cost-effective, as you only pay for the resources you consume, making it an economical choice for businesses.
Conclusion
The combined strengths of the vector engine for Amazon OpenSearch Serverless and Amazon Rekognition mark a new era of efficiency, cost-effectiveness, and heightened user satisfaction in intelligent and accurate digital media asset searches. This solution equips businesses with the tools to explore new possibilities, establishing itself as a vital asset for industries reliant on robust image management systems.
The benefits of this solution have been measured in these key areas:
- First, search efficiency has seen a remarkable 60% improvement. This translates into significantly enhanced user experiences, with clients and staff gaining swift and accurate access to the right images.
- Furthermore, the automated image metadata generation feature has slashed manual tagging efforts by a staggering 75%, resulting in substantial cost savings and freeing up valuable human resources. This not only guarantees data identification accuracy but also fosters consistency in asset management.
- In addition, the solution’s scalability has led to a 40% reduction in infrastructure costs. The serverless architecture permits cost-effective, on-demand scaling without the need for hefty hardware investments.
In summary, the fusion of the vector engine for Amazon OpenSearch Serverless and Amazon Rekognition for intelligent and accurate digital image search capabilities has proven to be a game-changer for businesses, especially for businesses seeking to leverage this type of solution to streamline and improve the utilization of their image repository for advertising, e-commerce, and content creation.
If you’re looking to modernize your cloud journey with AWS, and want to learn more about the serverless capabilities of Amazon OpenSearch Service, the vector engine, and other technologies, please contact us.
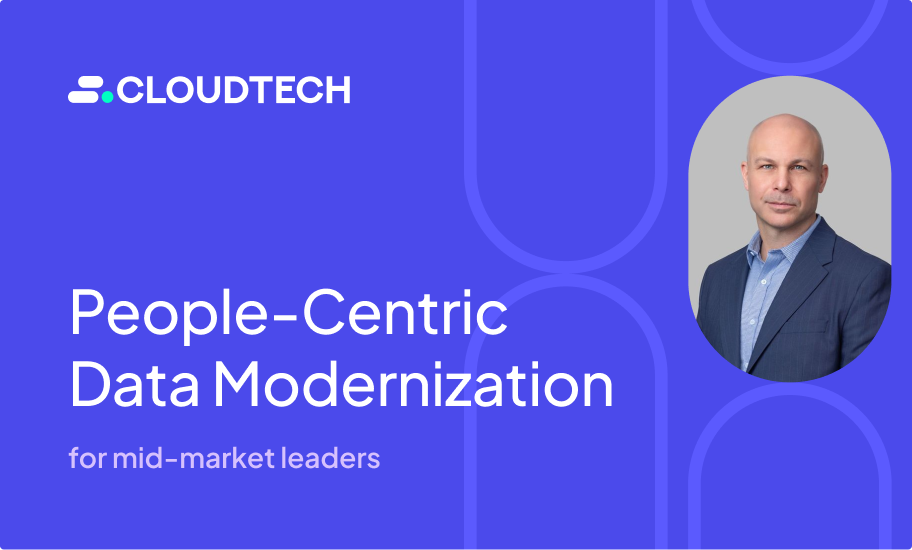
Cloudtech's Approach to People-Centric Data Modernization for Mid-Market Leaders
Engineering leaders at mid-market organizations are facing a significant challenge: managing complex data systems while juggling rapid growth and outdated technologies. Many of these organizations left their former on-premises ecosystems for greener pastures in the cloud without fully understanding the impact of this change. This shift has created issues from higher-than-expected cloud costs to engineers who are unprepared to turn over control to cloud providers. These new challenges are not only highly technical but also deeply human, affecting teams and processes at every level. Cloudtech understands these challenges and addresses them with a unique approach combining a people-centric focus paired with an iterative delivery mechanism ensuring transparency throughout the entire engagement.
Embracing a People-Centric Approach
At the heart of Cloudtech's philosophy is a simple truth: technology should serve people, not the other way around. This people-centric approach begins with a deep understanding of the needs, challenges, and capabilities of your team. Based on our findings, we are able to create customized solutions to fit your organization’s data infrastructure modernization goals. By focusing on this human aspect, Cloudtech ensures that our solutions don't just solve technical problems but also support and empower the people who spend their lives ensuring organizations have the right technology solutions to grow and support business needs.
Iterative, Tangible Improvements
Our “base hits” approach terminology comes from baseball, where base hits, though seemingly small, lead to significant victories. Cloudtech adopts a similar philosophy when we engage with our customers. This base hits approach is about consistent, manageable progress that accumulates over time. For your team, this means a sense of continuous achievement and motivation, allowing you to measure progress at every stage.
A Different Kind of Company
At Cloudtech, we are redefining the essence of professional services in the cloud industry. Our foundation is built on the expertise of engineering leaders who intimately understand the pressures of the role. Our mission is to assist mid-market companies to get the most out of their data, helping them centralize and modernize their data infrastructure to improve decision making and prepare them for a Generative AI future. We accomplish this by leveraging AWS serverless solutions to help our customers improve operational efficiency, reduce infrastructure management and lower their cloud costs.
Ready to get the most out of your data?
Discover how our unique delivery approach can streamline your processes, lower your cloud costs and help you feel confident about the current and future state of your organization’s technology.
Take the first step to modernizing your data infrastructure.
Schedule a Consultation | Learn More About Our Solutions
.jpeg)
Building Modern Data Streaming Architecture on AWS
What’s new in AWS Kinesis?
Amazon Kinesis Data firehose now also supports dynamic partitioning, where it continuously groups in transit data using dynamically or statically defined data keys and delivers the data into the individual amazon S3 prefixes by key. This reduces time to insight by minutes, it also reduces the cost, and overall simplifies the architecture.
Working with Streaming Data
For working with streaming data using Apache Flink, we also have AWS kinesis data analytics service, as with Amazon Kinesis Datastream like Kinesis Data Firehose, this service is also a fully managed, serverless, Apache Flink environment to perform stateful processing with sub-second latency. It integrates with several AWS services, supports custom connectors, and has a notebook interface called KDA Studio (Kinesis Data Analytics Studio), a managed Apache Zeppelin notebook, to allow you to interact with streaming data.
Similar to Kinesis Data Analytics for Apache Flink, Amazon managed stream for Apache Kafka or MSK is a fully managed service for running highly available, event-driven, Apache Kafka applications.
Amazon MSK operates, maintains, and scales Apache Kafka clusters, provides enterprises with security features and supports Kafka connect, and also has multiple built-in AWS integrations.
Architecture for Real-Time Reporting
Here we derive insights from input data that are coming from diverse sources or generating near real-time dashboards. With the below architecture what you are seeing is, that you can stream near real-time data from source systems such as social media applications using Amazon MSK, Lambda, and Kinesis Data Firehose into Amazon S3, you can then use AWS glue for Data Processing and Load, Transform data into Amazon redshift using an AWS glue developed endpoint such as an Amazon Sagemaker Notebook. Once data is in Amazon Redshift, you can create a customer-centric business report using Amazon Quick sight.
This architecture helps in identifying an act on deviation from the forecasted data in near real-time. In the below architecture, data is collected from multiple sources using Kinesis Data Stream, it is then persisted in Amazon S3 by Kenisis Data firehose, initial data aggregation, and preparation is done using Amazon Athena and then stored in the AWS S3. Amazon Sagemaker is used to train a forecasting model and create behavioral predictions. As new data arrives it is aggregated and prepared in real-time by Kinesis Data Analytics. The results are compared to the previously generated forecast, Amazon Cloud Watch is used to store the forecast and actual value as metrics, and when actual value deviates and cloud watch alarms trigger an incident in AWS Systems Manager, Incident manager.
Real-time reporting
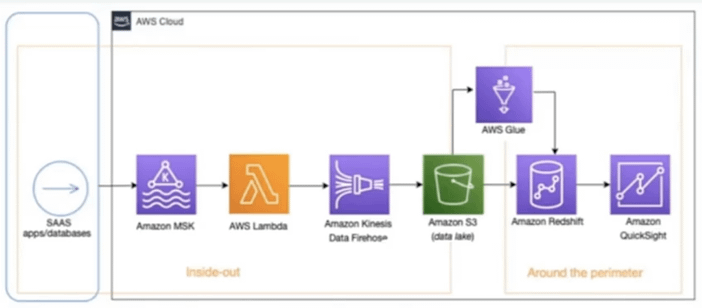
Architecture for Monitoring Streaming Data with Machine Learning
This architecture helps in identifying an act on deviation from the forecasted data in near real-time. In the below architecture, data is collected from multiple sources using Kinesis Data Stream, it is then persisted in Amazon S3 by Kenisis Data firehose, initial data aggregation, and preparation is done using Amazon Athena and then stored in the AWS S3. Amazon Sagemaker is used to train a forecasting model and create behavioral predictions. As new data arrives it is aggregated and prepared in real-time by Kinesis Data Analytics. The results are compared to the previously generated forecast, Amazon Cloud Watch is used to store the forecast and actual value as metrics, and when actual value deviates and cloud watch alarms trigger an incident in AWS Systems Manager, Incident manager.
Monitoring streaming data
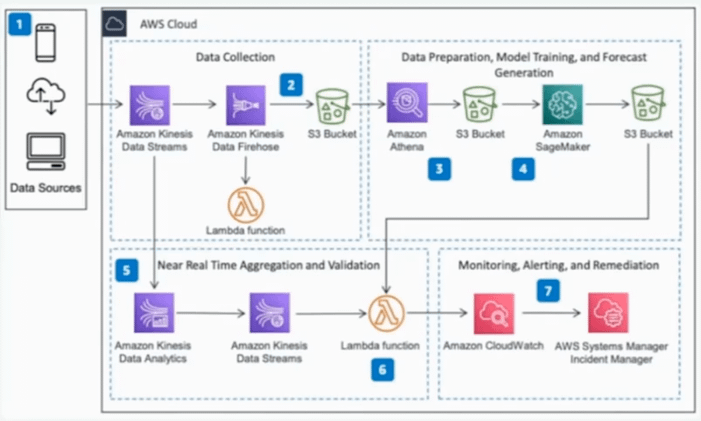
Conclusion
The key considerations, when working with AWS Streaming Services and Streaming Applications. When you need to choose a particular service or build a solution
Usage Patterns
Kinesis Data Stream is for collecting and storing data, and Kinesis Data Firehose is primarily for Loading and Transforming Data Streams into AWS Data Stores and Several Saas, endpoints. Kinesis Data Analytics essentially analyzes streaming data.
Throughput
Kinesis streams scale with shards and support up to 1Mb payloads, as mentioned earlier, you have a provisioning mode and an on-demand mode for scaling shard capacity. Kinesis firehose automatically scales to match the throughput of your data. The maximum streaming throughput a single Kinesis Data Analytics for SQL application can process is approximately 100 Mbps.
Latency
Kinesis Streams allows data delivery from producers to consumers in less than 70 milliseconds.
Ease of use and cost
All the streaming services on AWS are managed and serverless, including Amazon MSK serverless, this allows for ease of use by abstracting away the infrastructure management overhead and of course, considering the pricing model of each service for your unique use case.