What’s new in AWS Kinesis?
Amazon Kinesis Data firehose now also supports dynamic partitioning, where it continuously groups in transit data using dynamically or statically defined data keys and delivers the data into the individual amazon S3 prefixes by key. This reduces time to insight by minutes, it also reduces the cost, and overall simplifies the architecture.
Working with Streaming Data
For working with streaming data using Apache Flink, we also have AWS kinesis data analytics service, as with Amazon Kinesis Datastream like Kinesis Data Firehose, this service is also a fully managed, serverless, Apache Flink environment to perform stateful processing with sub-second latency. It integrates with several AWS services, supports custom connectors, and has a notebook interface called KDA Studio (Kinesis Data Analytics Studio), a managed Apache Zeppelin notebook, to allow you to interact with streaming data.
Similar to Kinesis Data Analytics for Apache Flink, Amazon managed stream for Apache Kafka or MSK is a fully managed service for running highly available, event-driven, Apache Kafka applications.
Amazon MSK operates, maintains, and scales Apache Kafka clusters, provides enterprises with security features and supports Kafka connect, and also has multiple built-in AWS integrations.
Architecture for Real-Time Reporting
Here we derive insights from input data that are coming from diverse sources or generating near real-time dashboards. With the below architecture what you are seeing is, that you can stream near real-time data from source systems such as social media applications using Amazon MSK, Lambda, and Kinesis Data Firehose into Amazon S3, you can then use AWS glue for Data Processing and Load, Transform data into Amazon redshift using an AWS glue developed endpoint such as an Amazon Sagemaker Notebook. Once data is in Amazon Redshift, you can create a customer-centric business report using Amazon Quick sight.
This architecture helps in identifying an act on deviation from the forecasted data in near real-time. In the below architecture, data is collected from multiple sources using Kinesis Data Stream, it is then persisted in Amazon S3 by Kenisis Data firehose, initial data aggregation, and preparation is done using Amazon Athena and then stored in the AWS S3. Amazon Sagemaker is used to train a forecasting model and create behavioral predictions. As new data arrives it is aggregated and prepared in real-time by Kinesis Data Analytics. The results are compared to the previously generated forecast, Amazon Cloud Watch is used to store the forecast and actual value as metrics, and when actual value deviates and cloud watch alarms trigger an incident in AWS Systems Manager, Incident manager.
Real-time reporting
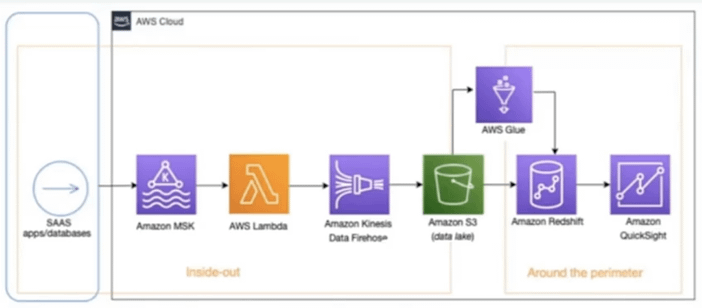
Architecture for Monitoring Streaming Data with Machine Learning
This architecture helps in identifying an act on deviation from the forecasted data in near real-time. In the below architecture, data is collected from multiple sources using Kinesis Data Stream, it is then persisted in Amazon S3 by Kenisis Data firehose, initial data aggregation, and preparation is done using Amazon Athena and then stored in the AWS S3. Amazon Sagemaker is used to train a forecasting model and create behavioral predictions. As new data arrives it is aggregated and prepared in real-time by Kinesis Data Analytics. The results are compared to the previously generated forecast, Amazon Cloud Watch is used to store the forecast and actual value as metrics, and when actual value deviates and cloud watch alarms trigger an incident in AWS Systems Manager, Incident manager.
Monitoring streaming data
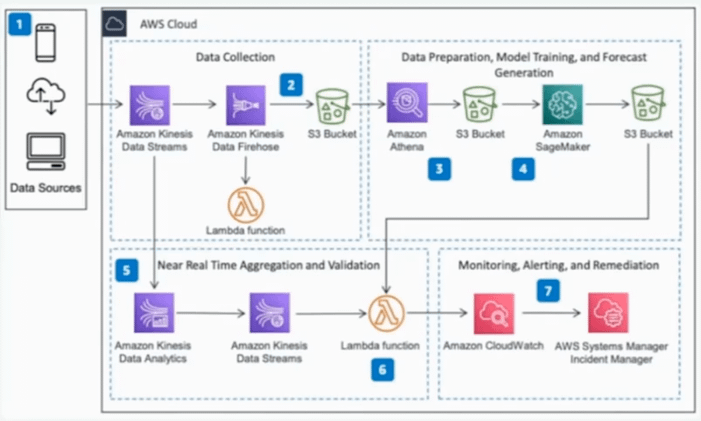
Conclusion
The key considerations, when working with AWS Streaming Services and Streaming Applications. When you need to choose a particular service or build a solution
Usage Patterns
Kinesis Data Stream is for collecting and storing data, and Kinesis Data Firehose is primarily for Loading and Transforming Data Streams into AWS Data Stores and Several Saas, endpoints. Kinesis Data Analytics essentially analyzes streaming data.
Throughput
Kinesis streams scale with shards and support up to 1Mb payloads, as mentioned earlier, you have a provisioning mode and an on-demand mode for scaling shard capacity. Kinesis firehose automatically scales to match the throughput of your data. The maximum streaming throughput a single Kinesis Data Analytics for SQL application can process is approximately 100 Mbps.
Latency
Kinesis Streams allows data delivery from producers to consumers in less than 70 milliseconds.
Ease of use and cost
All the streaming services on AWS are managed and serverless, including Amazon MSK serverless, this allows for ease of use by abstracting away the infrastructure management overhead and of course, considering the pricing model of each service for your unique use case.
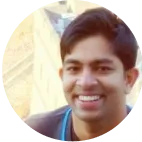
Building Modern Data Streaming Architecture on AWS
Related Resources
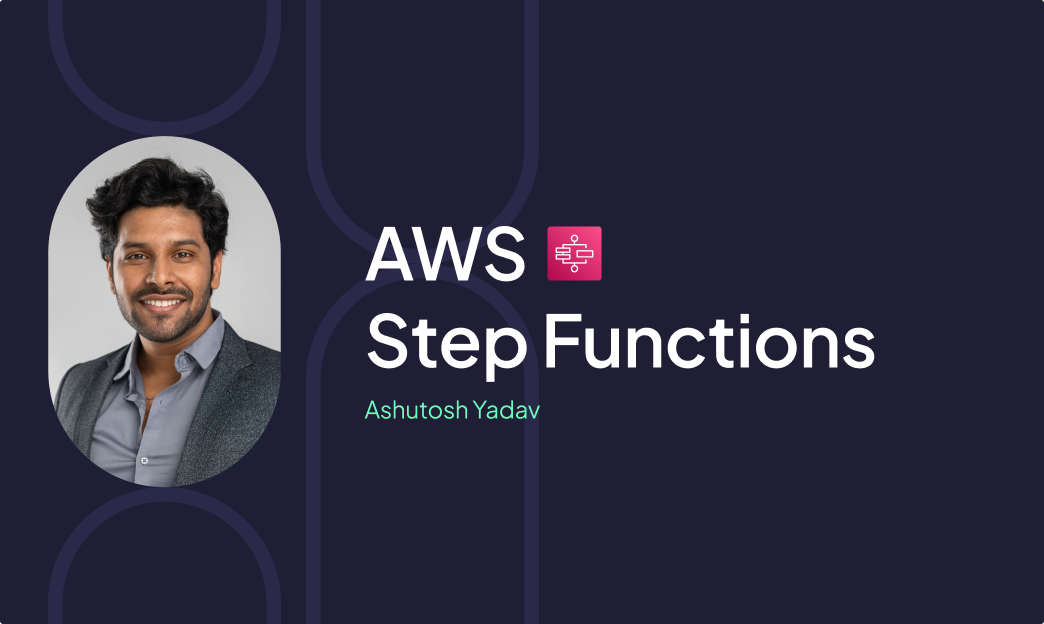
In the rapidly evolving cloud computing landscape, AWS Step Functions has emerged as a cornerstone for developers looking to orchestrate complex, distributed applications seamlessly in serverless implementations. The recent expansion of AWS SDK integrations marks a significant milestone, introducing support for 33 additional AWS services, including cutting-edge tools like Amazon Q, AWS B2B Data Interchange, AWS Bedrock, Amazon Neptune, and Amazon CloudFront KeyValueStore, etc. This enhancement not only broadens the horizon for application development but also opens new avenues for serverless data processing.
Serverless computing has revolutionized the way we build and scale applications, offering a way to execute code in response to events without the need to manage the underlying infrastructure. With the latest updates to AWS Step Functions, developers now have at their disposal a more extensive toolkit for creating serverless workflows that are not only scalable but also cost-efficient and less prone to errors.
In this blog, we will delve into the benefits and practical applications of these new integrations, with a special focus on serverless data processing. Whether you're managing massive datasets, streamlining business processes, or building real-time analytics solutions, the enhanced capabilities of AWS Step Functions can help you achieve more with less code. By leveraging these integrations, you can create workflows that directly invoke over 11,000+ API actions from more than 220 AWS services, simplifying the architecture and accelerating development cycles.
Practical Applications in Data Processing:
This AWS SDK integration with 33 new services not only broadens the scope of potential applications within the AWS ecosystem but also streamlines the execution of a wide range of data processing tasks. These integrations empower businesses with automated AI-driven data processing, streamlined EDI document handling, and enhanced content delivery performance.
Amazon Q Integration: Amazon Q is a generative AI-powered enterprise chat assistant designed to enhance employee productivity in various business operations. The integration of Amazon Q with AWS Step Functions enhances workflow automation by leveraging AI-driven data processing. This integration allows for efficient knowledge discovery, summarization, and content generation across various business operations. It enables quick and intuitive data analysis and visualization, particularly beneficial for business intelligence. In customer service, it provides real-time, data-driven solutions, improving efficiency and accuracy. It also offers insightful responses to complex queries, facilitating data-informed decision-making.
AWS B2B Data Interchange: Integrating AWS B2B Data Interchange with AWS Step Functions streamlines and automates electronic data interchange (EDI) document processing in business workflows. This integration allows for efficient handling of transactions including order fulfillment and claims processing. The low-code approach simplifies EDI onboarding, enabling businesses to utilize processed data in applications and analytics quickly. This results in improved management of trading partner relationships and real-time integration with data lakes, enhancing data accessibility for analysis. The detailed logging feature aids in error detection and provides valuable transaction insights, essential for managing business disruptions and risks.
Amazon CloudFront KeyValueStore: This integration enhances content delivery networks by providing fast, reliable access to data across global networks. It's particularly beneficial for businesses that require quick access to large volumes of data distributed worldwide, ensuring that the data is always available where and when it's needed.
Neptune Data: This integration allows the Processing of graph data in a serverless environment, ideal for applications that require complex relationships and data patterns like social networks, recommendation engines, and knowledge graphs. For instance, Step Functions can orchestrate a series of tasks that ingest data into Neptune, execute graph queries, analyze the results, and then trigger other services based on those results, such as updating a dashboard or triggering alerts.
Amazon Timestream Query & Write: The integration is useful in serverless architectures for analyzing high-volume time-series data in real-time, such as sensor data, application logs, and financial transactions. Step Functions can manage the flow of data from ingestion (using Timestream Write) to analysis (using Timestream Query), including data transformation, anomaly detection, and triggering actions based on analytical insights.
Amazon Bedrock & Bedrock Runtime: AWS Step Functions can orchestrate complex data streaming and processing pipelines that ingest data in real-time, perform transformations, and route data to various analytics tools or storage systems. Step Functions can manage the flow of data across different Bedrock tasks, handling error retries, and parallel processing efficiently
AWS Elemental MediaPackage V2: Step Functions can orchestrate video processing workflows that package, encrypt, and deliver video content, including invoking MediaPackage V2 actions to prepare video streams, monitoring encoding jobs, and updating databases or notification systems upon completion.
AWS Data Exports: With Step Functions, you can sequence tasks such as triggering data export actions, monitoring their progress, and executing subsequent data processing or notification steps upon completion. It can automate data export workflows that aggregate data from various sources, transform it, and then export it to a data lake or warehouse.
Benefits of the New Integrations
The recent integrations within AWS Step Functions bring forth a multitude of benefits that collectively enhance the efficiency, scalability, and reliability of data processing and workflow management systems. These advancements simplify the architectural complexity, reduce the necessity for custom code, and ensure cost efficiency, thereby addressing some of the most pressing challenges in modern data processing practices. Here's a summary of the key benefits:
Simplified Architecture: The new service integrations streamline the architecture of data processing systems, reducing the need for complex orchestration and manual intervention.
Reduced Code Requirement: With a broader range of integrations, less custom code is needed, facilitating faster deployment, lower development costs, and reduced error rates.
Cost Efficiency: By optimizing workflows and reducing the need for additional resources or complex infrastructure, these integrations can lead to significant cost savings.
Enhanced Scalability: The integrations allow systems to easily scale, accommodating increasing data loads and complex processing requirements without the need for extensive reconfiguration.
Improved Data Management: These integrations offer better control and management of data flows, enabling more efficient data processing, storage, and retrieval.
Increased Flexibility: With a wide range of services now integrated with AWS Step Functions, businesses have more options to tailor their workflows to specific needs, increasing overall system flexibility.
Faster Time-to-Insight: The streamlined processes enabled by these integrations allow for quicker data processing, leading to faster time-to-insight and decision-making.
Enhanced Security and Compliance: Integrating with AWS services ensures adherence to high security and compliance standards, which is essential for sensitive data processing and regulatory requirements.
Easier Integration with Existing Systems: These new integrations make it simpler to connect AWS Step Functions with existing systems and services, allowing for smoother digital transformation initiatives.
Global Reach: Services like Amazon CloudFront KeyValueStore enhance global data accessibility, ensuring high performance across geographical locations.
As businesses continue to navigate the challenges of digital transformation, these new AWS Step Functions integrations offer powerful solutions to streamline operations, enhance data processing capabilities, and drive innovation. At Cloudtech, we specialize in serverless data processing and event-driven architectures. Contact us today and ask how you can realize the benefits of these new AWS Step Functions integrations in your data architecture.
In today's digital landscape, user experience is paramount, and search engines play a pivotal role in shaping it. Imagine a world where your search engine not only understands your preferences and needs but anticipates them, delivering results that resonate with you on a personal level. This transformative user experience is made possible by the fusion of Amazon Personalize and Amazon OpenSearch Service.
Understanding Amazon Personalize
Amazon Personalize is a fully-managed machine learning service that empowers businesses to develop and deploy personalized recommendation systems, search engines, and content recommendation engines. It is part of the AWS suite of services and can be seamlessly integrated into web applications, mobile apps, and other digital platforms.
Key components and features of Amazon Personalize include:
Datasets: Users can import their own data, including user interaction data, item data, and demographic data, to train the machine learning models.
Recipes: Recipes are predefined machine learning algorithms and models that are designed for specific use cases, such as personalized product recommendations, personalized search results, or content recommendations.
Customization: Users have the flexibility to fine-tune and customize their machine learning models, allowing them to align the recommendations with their specific business goals and user preferences.
Real-Time Recommendations: Amazon Personalize can generate real-time recommendations for users based on their current behavior and interactions.
Batch Recommendations: Businesses can also generate batch recommendations for users, making it suitable for email campaigns, content recommendations, and more.
Benefits of Amazon Personalize
Amazon Personalize offers a range of benefits for businesses looking to enhance user experiences and drive engagement.
Improved User Engagement: By providing users with personalized content and recommendations, Amazon Personalize can significantly increase user engagement rates.
Higher Conversion Rates: Personalized recommendations often lead to higher conversion rates, as users are more likely to make purchases or engage with desired actions when presented with items or content tailored to their preferences.
Enhanced User Satisfaction: Personalization makes users feel understood and valued, leading to improved satisfaction with your platform. Satisfied users are more likely to become loyal customers.
Better Click-Through Rates (CTR): Personalized recommendations and search results can drive higher CTR as users are drawn to content that aligns with their interests, increasing their likelihood of clicking through to explore further.
Increased Revenue: The improved user engagement and conversion rates driven by Amazon Personalize can help cross-sell and upsell products or services effectively.
Efficient Content Discovery: Users can easily discover relevant content, products, or services, reducing the time and effort required to find what they are looking for.
Data-Driven Decision Making: Amazon Personalize provides valuable insights into user behavior and preferences, enabling businesses to make data-driven decisions and optimize their offerings.
Scalability: As an AWS service, Amazon Personalize is highly-scalable and can accommodate businesses of all sizes, from startups to large enterprises.
Understanding Amazon OpenSearch Service
Amazon OpenSearch Service is a fully managed, open-source search and analytics engine developed to provide fast, scalable, and highly-relevant search results and analytics capabilities. It is based on the open-source Elasticsearch and Kibana projects and is designed to efficiently index, store, and search through vast amounts of data.
Benefits of Amazon OpenSearch Service in Search Enhancement
Amazon OpenSearch Service enhances search functionality in several ways:
High-Performance Search: OpenSearch Service enables organizations to rapidly execute complex queries on large datasets to deliver a responsive and seamless search experience.
Scalability: OpenSearch Service is designed to be horizontally scalable, allowing organizations to expand their search clusters as data and query loads increase, ensuring consistent search performance.
Relevance and Ranking: OpenSearch Service allows developers to customize ranking algorithms to ensure that the most relevant search results are presented to users.
Full-Text Search: OpenSearch Service excels in full-text search, making it well-suited for applications that require searching through text-heavy content such as documents, articles, logs, and more. It supports advanced text analysis and search features, including stemming and synonym matching.
Faceted Search: OpenSearch Service supports faceted search, enabling users to filter search results based on various attributes, categories, or metadata.
Analytics and Insights: Beyond search, OpenSearch Service offers analytics capabilities, allowing organizations to gain valuable insights into user behavior, query performance, and data trends to inform data-driven decisions and optimizations.
Security: OpenSearch Service offers access control, encryption, and authentication mechanisms to safeguard sensitive data and ensure secure search operations.
Open-Source Compatibility: While Amazon OpenSearch Service is a managed service, it remains compatible with open-source Elasticsearch, ensuring that organizations can leverage their existing Elasticsearch skills and applications.
Integration Flexibility: OpenSearch Service can seamlessly integrate with various AWS services and third-party tools, enabling organizations to ingest data from multiple sources and build comprehensive search solutions.
Managed Service: Amazon OpenSearch Service is a fully-managed service, which means AWS handles the operational aspects, such as cluster provisioning, maintenance, and scaling, allowing organizations to focus on developing applications and improving user experiences.
Amazon Personalize and Amazon OpenSearch Service Integration
When you use Amazon Personalize with Amazon OpenSearch Service, Amazon Personalize re-ranks OpenSearch Service results based on a user's past behavior, any metadata about the items, and any metadata about the user. OpenSearch Service then incorporates the re-ranking before returning the search response to your application. You control how much weight OpenSearch Service gives the ranking from Amazon Personalize when applying it to OpenSearch Service results.
With this re-ranking, results can be more engaging and relevant to a user's interests. This can lead to an increase in the click-through rate and conversion rate for your application. For example, you might have an ecommerce application that sells cars. If your user enters a query for Toyota cars and you don't personalize results, OpenSearch Service would return a list of cars made by Toyota based on keywords in your data. This list would be ranked in the same order for all users. However, if you were to use Amazon Personalize, OpenSearch Service would re-rank these cars in order of relevance for the specific user based on their behavior so that the car that the user is most likely to click is ranked first.
When you personalize OpenSearch Service results, you control how much weight (emphasis) OpenSearch Service gives the ranking from Amazon Personalize to deliver the most relevant results. For instance, if a user searches for a specific type of car from a specific year (such as a 2008 Toyota Prius), you might want to put more emphasis on the original ranking from OpenSearch Service than from Personalize. However, for more generic queries that result in a wide range of results (such as a search for all Toyota vehicles), you might put a high emphasis on personalization. This way, the cars at the top of the list are more relevant to the particular user.
How the Amazon Personalize Search Ranking plugin works
The following diagram shows how the Amazon Personalize Search Ranking plugin works.

- You submit your customer's query to your Amazon OpenSearch Service Cluster
- OpenSearch Service sends the query response and the user's ID to the Amazon Personalize search ranking plugin.
- The plugin sends the items and user information to your Amazon Personalize campaign for ranking. It uses the recipe and campaign Amazon Resource Name (ARN) values within your search process to generate a personalized ranking for the user. This is done using the GetPersonalizedRanking API operation for recommendations. The user's ID and the items obtained from the OpenSearch Service query are included in the request.
- Amazon Personalize returns the re-ranked results to the plugin.
- The plugin organizes and returns these search results to your OpenSearch Service cluster. It re-ranks the results based on the feedback from your Amazon Personalize campaign and the emphasis on personalization that you've defined during setup.
- Finally, your OpenSearch Service cluster sends the finalized results back to your application.
Benefits of Amazon Personalize and Amazon OpenSearch Service Integration
Combining Amazon Personalize and Amazon OpenSearch Service maximizes user satisfaction through highly personalized search experiences:
Enhanced Relevance: The integration ensures that search results are tailored precisely to individual user preferences and behavior. Users are more likely to find what they are looking for quickly, resulting in a higher level of satisfaction.
Personalized Recommendations: Amazon Personalize's machine learning capabilities enable the generation of personalized recommendations within search results. This feature exposes users to items or content they may not have discovered otherwise, enriching their search experience.
User-Centric Experience: Personalized search results demonstrate that your platform understands and caters to each user's unique needs and preferences. This fosters a sense of appreciation and enhances user satisfaction.
Time Efficiency: Users can efficiently discover relevant content or products, saving time and effort in the search process.
Reduced Information Overload: Personalized search results also filter out irrelevant items to reduce information overload, making decision-making easier and more enjoyable.
Increased Engagement: Users are more likely to engage with content or products that resonate with their interests, leading to longer session durations and a greater likelihood of conversions.
Conclusion
Integrating Amazon Personalize and Amazon OpenSearch Service transforms user experiences, drives user engagement, and unlocks new growth opportunities for your platform or application. By embracing this innovative combination and encouraging its adoption, you can lead the way in delivering exceptional personalized search experiences in the digital age.
%2520dark.jpeg)
Irrespective of your business size, you can’t ignore the value assessment of any product or service you plan to purchase. The right investment in the right system, process, and infrastructure is essential for success in your business. And how can you make the right financial decisions and understand whether “X” product/service is generating value or not for your business?
Total Cost of Ownership or TCO analysis is one method that can help you in this situation, especially if you are planning to analyze these costs on the cloud. Amazon’s AWS is a leading public cloud platform offering over 200 fully featured services, including AWS TCO analysis -a service to analyze the total costs of an asset or infrastructure on the cloud. It offers services to diverse customers – startups, government agencies, and the largest enterprises. Its agility, innovation, safety, and several data centers make it comprehensive and adaptable.
Read on to learn more about the TCO analysis and how AWS TCO analysis works.
What is TCO analysis?
As the name suggests, TCO estimates costs associated with purchasing, deploying, operating, and maintaining any asset. The asset could be physical or virtual products, services, or tools. The TCO analysis’s primary purpose is to assess the asset’s cost throughout its life cycle and to determine the return on investment.
Regarding the IT industry, TCO analysis consists of costs related to hardware/software acquisition, end-user expenses, training, network, servers, and communications. According to Gartner, “TCO is a comprehensive assessment of IT or other costs across enterprise boundaries over time.”
TCO analysis in Cloud
The adoption of cloud computing in business also raises the trend of TCO analysis on the cloud. You can call it, cloud TCO analysis, which performs the same job on the cloud. TCO analysis in the cloud calculated the total costs of adopting, executing, and provisioning cloud infrastructure. When you are planning to migrate to the cloud, this analysis helps you to weigh the current costs and cloud adoption costs. Not only Amazon, but other big tech giants, including Microsoft, Google, IBM, and many more, offering TCO analysis in the cloud. But, Amazon’s AWS is the number one cloud service provider to offer cloud services.
Why do businesses need AWS TCO analysis?
A TCO analysis helps to know whether there will be profit or loss.
Let’s understand it with an example showing how AWS TCO analysis helped the company increase its profit. The top OTT platform, Netflix, invested $9.6 million per month in AWS Cost in 2019, which would increase by 2023. According to this resource, it would be around $27.78 million per month. The biggest reason behind this investment is the profit, and AWS TCO analysis is helping them to know how this profit is happening. AWS helped Netflix to get a cost-effective and scalable cloud architecture horizontally. It also enabled the company to focus on its core business – video streaming services. You all know that Netflix is the favorite video streaming platform globally.
In another example, delaying the decision of TCO analysis ignorance resulted in a loss. According to this report on 5GC, TCO analysis has been done regarding the adoption of the 5G core. It has been found that postponing increases the TCO over five years. It indicates the losses occurred due to ignorance of TCO analysis.
These examples show that your business needs both TCO analysis and cloud infrastructure. A lack of TCO analysis might cause incorrect IT budget calculations or purchasing of inappropriate resources. It might result in problems like downtime and slower business operations. You can understand that the TCO analysis is a critical business operation. Its ignorance directly impacts financial decisions. Thus, know this and utilize AWS TCO analysis for your business success.
How does AWS TCO Analysis work?
AWS TCO analysis refers to calculating the direct and indirect costs associated with migrating, hosting, running, and maintaining IT infrastructure on the AWS cloud. It assesses all the costs of using AWS resources and compares the outcome to the TCO of an alternative cloud or on-premises platform.
AWS TCO Analysis is not a calculation of one resource or a one-step process. To understand how it works, you need to know the costs of your current IT infrastructure, understand cost factors, and how to optimize cloud costs when you deploy and manage scalable web applications or infrastructure hosted on-premises versus when you deploy them on the cloud.
Here are steps to help you understand how AWS TCO analysis works:
Preliminary steps – Know the current value and build a strategy
Step 1 – Evaluate your existing infrastructure/ web application cost
You must calculate and analyze the direct and indirect costs of your existing on-premise IT infrastructure. Perform the TCO analysis of this infrastructure, including various components.
- Physical & virtual servers: They are the main pillars in developing the infrastructure
- Storage mediums: Cost of database, disks, or other storage devices
- Software & Applications: The analysis finds the cost of software and its constant upgrades. It also estimates the costs of acquiring licenses, subscriptions, loyalties, and vendor fees
- Data centers: The analysis needs to check the costs of all linked equipment such as physical space, power, cooking, and racks with the data centers
- Human Capital: Trainers, consultants, and people who run setups.
- Networking & Security system: Find out the costs of these critical components
Don’t limit yourself to estimating only direct/indirect costs. Find out any hidden costs that might happen due to unplanned events like downtime and opportunity costs, which might be helpful in the future.
Step 2 – Build an appropriate cloud migration strategy
You must choose an appropriate AWS cloud migration strategy before calculating monthly AWS costs. Amazon offers many TCO analysis migration tools, such as CloudChomp CC Analyzer, Cloudamize, Migration Evaluators, etc., from AWS and AWS partners. It can help you to evaluate the existing environment, determine workloads, and plan the AWS migration. It provides excellent insights regarding the costs, which can help you to make quick and effective decisions for migration to AWS.
Primary step – Estimate AWS Cost
Know these cost factors
All industries have different objectives and business operations. Thus, their cost analysis differs according to AWS services, workloads, servers, or methods of purchasing other AWS resources., the cost depends on the working usage of services and resources.
Still, you must consider the following factors directly impacting your AWS costs.
- Services you utilize: AWS offers various computing services, resources, and instances with hourly charges. It will bill you from when you launch any resource/instance until termination. You will get other options to use predetermined set costs for making reservations.
- Data Transfer: AWS charges for aggregated outbound data transfer across services according to a set rate. AWS does not charge for inbound or inter-service data transfer within a specific region. Still, you must check data transfer costs before launching.
- Storage: AWS charges for each GB of data storage. As per consumed storage classes, you need to understand the cost analysis. Remember that cold and hot storage options are available, but hot storage is expensive and accessible.
- Resource consumption model: You get options to consume resources. Such as on-demand instances, reserved instances that give discounted prepay options for on-demand instances, and AWS saving plans.
Know how to use AWS Pricing Calculator
Once you analyze your compute resources and infrastructure to deploy, understand these factors, and decide on necessary AWS resources, you need to use AWS Pricing Calculator for expected cost estimation. This tool helps determine the total ownership cost. It is a web service that is freely available to end-users. It permits you to explore services according to need and estimate costs.
Look at the below image to see how this calculator works. You have to add required services, configure them by providing details, and see the generated costs.
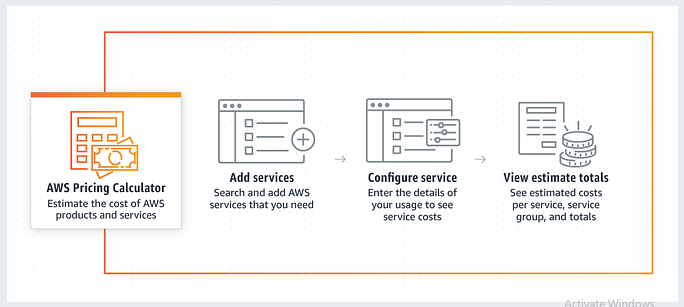
Credit: Amazon AWS Pricing Calculator
You can easily add the prices according to a group of services or individual services. After adding to the calculator, check the following snap-shot of the configuration service (EC2 service). You have to provide all required information such as location type, operating system, instance type, memory, pricing models, storage, and many more.

Credit: Amazon AWS Pricing Calculator
The best part is that you can download and share the results for further analysis. The following image is a dummy report to know that you can estimate the monthly cost, budget, and other factors with this summary.

Credit: Amazon AWS Pricing Calculator
Note: Check this link to know various factors for pricing assumptions.
Know how to optimize cloud costs on AWS
Calculation on AWS is not sufficient; you need to optimize your cost estimation. AWS offers various cost optimization options to manage, monitor, and optimize costs. Here are some tools you can utilize to optimize your costs on AWS:
Tool name | Key Characteristics |
AWS Trusted Advisor |
|
AWS Cost Explorer |
|
AWS Budgets |
|
AWS Costs & Usages Report |
|
Tool nameKey CharacteristicsAWS Trusted Advisor
- Get recommendations from this tool to follow the AWS best practices to improve performance, security, & fault tolerance
- Can help you to optimize your cloud deployment through context-driven recommendation
AWS Cost Explorer
- Provide you with an interface to check, visualize, and manage AWS costs and usages over time
- Features like filtering, grouping, and reporting can help you to manage costs efficiently
AWS Budgets
- Use this tool to track your costs and improve them for better budget planning and controlling
- You can also create custom actions that help prevent overages, inefficient resource usage, or lack of coverage
AWS Costs & Usages Report
- Leverage this tool to track your savings, costs, and cost drivers.
- You can easily integrate this report with an analytics report to get deep analysis
- It can help you to learn cost anomalies and trends in your bills
How Airbnb used AWS Cost & Usage Report for AWS cost optimization
A community marketplace, Airbnb, based in San Francisco founded in 2008. The community has over 7 million accommodations and over 40,000 customers. In 2016, Airbnb decided to migrate all operations to AWS to scale their infrastructure automatically. It worked, and in just 3 years, the company grew significantly and reduced its expenses through different AWS services (Amazon EC2, Amazon S3, Amazon EMR, etc). In 2021, the company utilized the tools, AWS cost & usage report, saving plans, and actional data to optimize their AWS costs. The outcome: 27% reduced storage costs; 60% reduced Amazon Open Search Service cost.
The company has developed a customized cost and usage data tool through AWS services. It is helping them to reduce costs and deliver actional business metrics.
Final Stage: Avoid these mistakes
Often, businesses make mistakes like misconfiguration, choosing the wrong resource, etc., leading to increased costs. Check the following points to avoid mistakes:
- Never create or set up cloud resources without using auto-scaling options or other monitoring tools. It happens during Dev/test environments mostly.
- Take care while configuring storage resources, classes, and data types. Often, misconfiguration happens during storage tiers usage, such as Simple Storage Service (S3).
- Avoid over-provisioned resources by properly consolidating them. You must know the concept of right-sizing to find the perfect match between instance types, sizes, and capacity requirements at a minimal cost.
- Choose a pricing plan carefully based on your infrastructure requirements. This mistake can cost you an expensive cloud deployment.
- Never ignore the newer technologies, as they can reduce your cloud spending and helps in increasing productivity in work.
Closing Thought
The report is proof to know that AWS helps businesses in cost savings up to 80% over the equivalent on-premises options. It lowers costs and allows companies to use savings for innovation. So, what are you waiting for, plan to migrate your on-premise IT infrastructure to the AWS cloud, calculate costs by following the steps, and optimize it by preventing typical mistakes?
Managing your IT infrastructure’s overall direct and indirect costs requires time and process. TCO analysis for a cloud migration project is a daunting job. But, AWS TCO analysis makes this complex process easy. Take advantage of this analysis and determine your cloud migration project cost.
Get started on your cloud modernization journey today!
Let Cloudtech build a modern AWS infrastructure that’s right for your business.